Direct-coupling analysis of residue coevolution captures native contacts across many protein families
BackGround
It has long been suggested that the resulting correlations among amino acid compositions at different sequence positions can be exploited to infer spatial contacts within the tertiary protein structure.
Crucial to this inference is the ability to disentangle direct and indirect correlations, as accomplished by the recently introduced direct-coupling analysis (DCA).
Here we develop a computationally efficient implementation of DCA, which allows us to evaluate the accuracy of contact prediction by DCA for a large number of protein domains, based purely on sequence information.
Here we will introduce mfDCA, an algorithm based on the mean-field approximation of DCA.
Methods
Data Extraction
For each family, the protein sequences are collected in one MSA denoted by
Sequence Statistics and Reweighting
The main inputs of DCA are reweighted frequency counts for single MSA columns and column pairs
重加权目的是为了纠正采样偏差,定义加权因子为
定义有效序列数
定义频率公式:
- 表示
在所有MSA的i列出现的频率 - 表示
在所有MSA的i列且 在j列出现的联合频率
其中
此时我们可以直接计算出互信息MI(根据信息熵公式):
但是文中认为MI表示直接与间接相关性效果不太好,我们可以通过后续来推出更好的指标DI
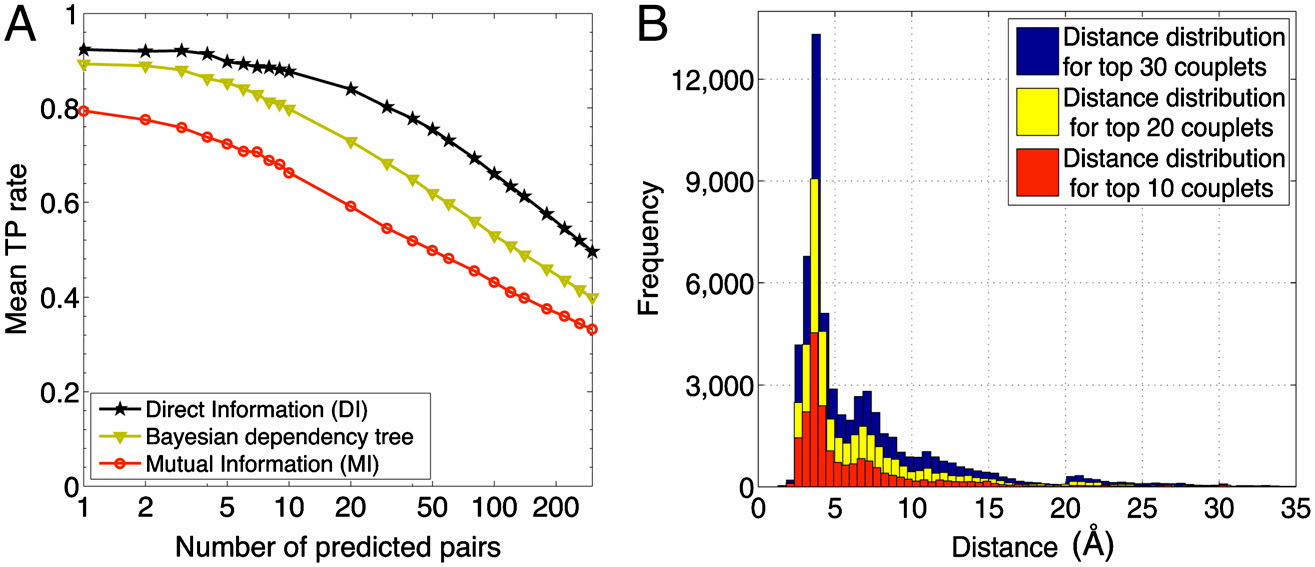
待更新